Changing human behavior with AI: How machine learning can automate motivational interviewing
Motivational interviewing (MI) is a strategy used by clinical psychologists to elicit behavior change in patients through focused, goal-oriented counseling. An integral step in developing effective MI interventions is analysis of the counselor-patient interactions, which is traditionally done by hand.
"Analyzing sequences of assigned behavior codes allows clinicians to better understand the patient's thought process during the course of the interviews, without having to wade through entire transcripts over and over again," said Alex Kotov, assistant professor of computer science at Wayne State University. "Such understanding, in turn, leads to further specification of the mechanisms of effect for behavioral intervention models, which can then be used to refine theory and guide clinical practice."
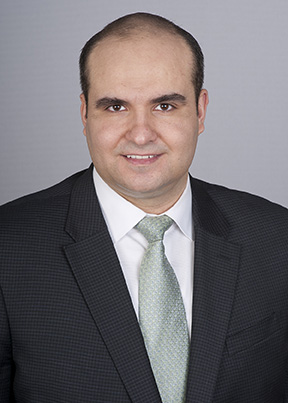
As manual analysis is both time consuming and labor-intensive, researchers have begun investigating machine learning techniques to find an efficient alternative.
Kotov teamed up with Gwen Alexander, from Henry Ford Health System, and April Carcone, an assistant professor in the WSU School of Medicine, in a collaborative effort to automate behavioral coding to advance MI's effect on patients. This work has been funded by the National Institutes of Health.
The team developed a system to automate segmentation of exchanges in e-coaching sessions, or behavioral counseling using e-mail or other electronic platforms, into communication behaviors and assignment of behavior codes to identified segments. It also proposed an algorithm to identify sequences and patterns in successful and unsuccessful sessions, leading to a greater understanding of effective MI strategies.
One specific type of e-coaching on which the team focused was with adolescent patients with unhealthy eating habits that lead to obesity or other health issues. However, Kotov notes that this technology can be applied much more broadly to other types of MI.
"We are aiming to help patients with a wide spectrum of behavioral disorders," said Kotov. "The goal is to determine which strategies work best and automate the entire MI process."
The experimental results were comparable to those obtained with human coders, demonstrating the promise of ML methods for more efficient behavioral coding and analysis. Kotov's plan for the future is to develop a system for fully-automated analysis and real-time monitoring of MI sessions.
This article originally appeared in the Fall 2018 edition of Exemplar.